안녕하세요, 오늘 읽은 논문은 DilatedNet, Multi-Scale Context Aggregation by Dilated Convolutions 입니다.
DilatedNet은 segmetation task를 위한 dilated convolution을 사용합니다. dilated convolution은 이미지의 해상도를 낮추지 않고, receptive field를 확장할 수 있습니다. 예를 들어, 기존의 CNN은 max pooling 연산 또는 stride=2인 conv 연산을 통하여 feature map의 크기를 축소한 뒤에 conv 연산을 적용하여 receptive field를 확장합니다. dilated conv는 이과정을 거치지 않아 공간적인 정보 훼손 없이 receptive field를 확장할 수 있습니다. 또한 Context module과 Front End module을 제안합니다.
정리하자면 DilatedNet은 dilated convolution으로 구성된 Context module을 사용하며, front-end module과 context module로 이루어져 있습니다. front-end module은 backnone으로 이해할 수 있으며, front end module이 생성한 feature map을 context module에 전달합니다.
DilatedNet 등장 배경
DilatedNet은 Dense prediction을 위해 고안되었습니다. Dense prediction은 segmentation같은 task를 의미합니다. classification과 다르게 segmentation은 각 카테고리 중 하나로 각 픽셀을 분류해야 합니다. classification에서 CNN이 좋은 성능을 달성할 수 있었던 이유는 CNN의 translation invariant 속성 덕분이었습니다. 이미지 내의 객체의 존재만 판단하면 되므로 정확한 위치 정보보다 모호한 위치 정보가 필요하여 translation invariant 속성이 필요했습니다. 하지만 localization이 필요한 segmentation과 object detection task에서는 translation invariant 속성이 성능에 악 영향을 주며, 이 이동 불변성을 깨려는 노력이 있습니다.
Dilated Convolutions
DilatedNet의 핵심 아이디어는 Dilated Convolution 입니다. dilated convolution은 resolution 손실 없이 receptive field를 확장할 수 있으며, receptive field가 확장되어도 파라미터 수가 증가하지 않습니다.
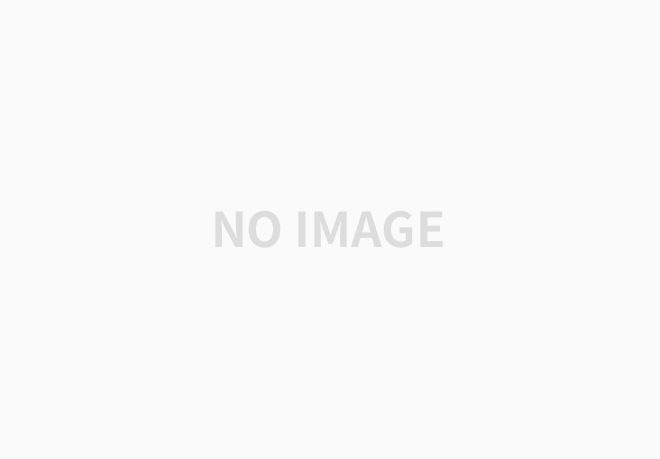
(a)의 filter size는 3x3이며, 파라미터는 9개를 갖고 있습니다. (b)는 dilated factor=2인 경우입니다. 빨간점을 제외한 filter의 가중치는 0으로 채워져 있어 9개의 파라미터를 갖으면서 receptive field는 7x7입니다. 즉, maxpooling이나 stride=2 conv를 적용하지 않아도 receptive field를 확장할 수 있어 공간 정보 훼손을 감소할 수 있고, 연산량을 유지하며 receptive field를 확장할 수 있는 이점이 있습니다.
pytorch로 dilated conv를 구현하는 경우에 nn.Conv2d에서 dilated 인자에 값을 추가하면 됩니다.
Multi-Scale Context Aggregation
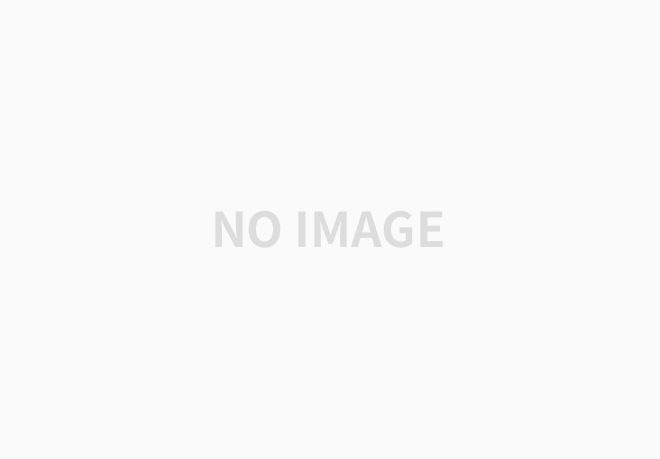
DilatedNet은 context module을 제안합니다. context module은 multi-scale contextual information 정보를 종합하여 dense prediction의 성능을 향상시킵니다. 이 모듈의 특징은 C채널의 feature map을 입력 받으면, C 채널의 feature map을 출력합니다. input과 output의 크기와 채널수가 동일하므로 다른 dense prediction 구조에 사용할 수 있습니다. 8 layer의 dilated conv로 이루어져 있습니다. truncated 는 conv 연산 이후에 ReLU를 사용하는 것을 의미합니다.
Front End
front end 모듈은 context module의 앞단에 연결되어 있는 일종의 backbone 입니다. 3 채널의 이미지를 입력 받아 C=21의 feature map을 출력합니다. VGG-16 net을 사용하며 마지막 두 pooling 연산을 제거하여 output 크기가 64x64가 되도록 합니다. 또한 conv block 5의 convolution을 모두 2-dilated convolution으로 변경합니다. conv block 5 뒤의 첫 fc_1을 4-dilated convolution으로 변경합니다. 3 채널의 입력 이미지를 입력 받아 21 채널의 64x64 feature map을 생성하고, 생성된 feature map을 context module로 전달합니다.
Performance
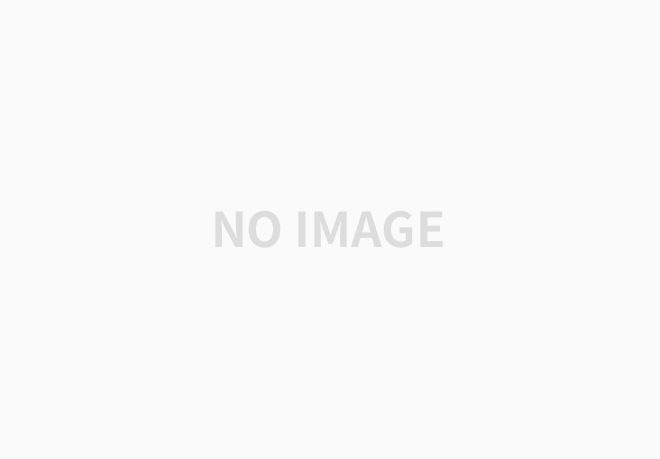
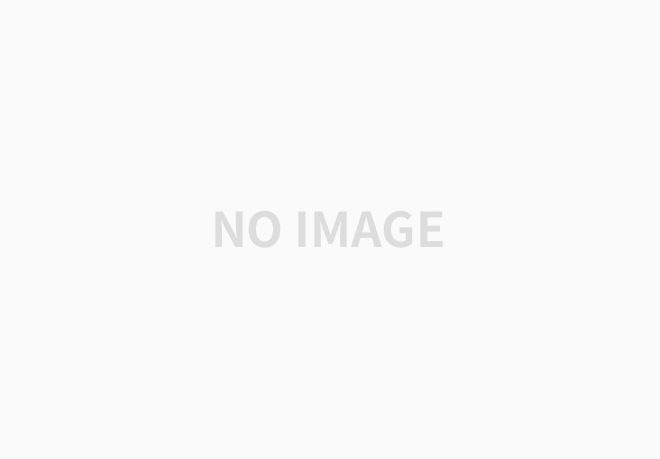
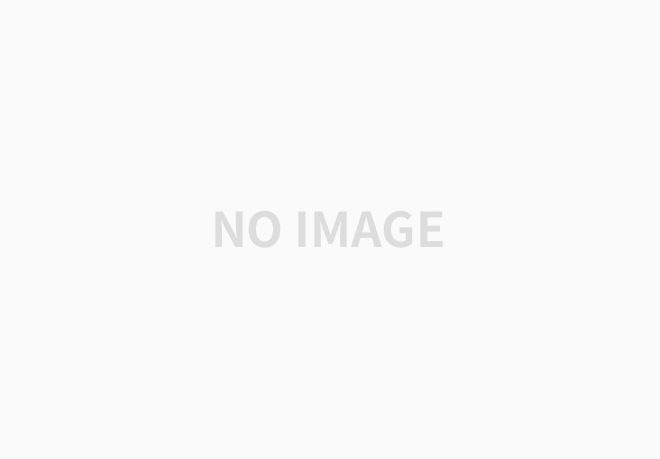
참고자료