Dense Contrastive Learning for Self-Supervised Visual Pre-Training
https://arxiv.org/abs/2011.09157
Dense Contrastive Learning for Self-Supervised Visual Pre-Training
To date, most existing self-supervised learning methods are designed and optimized for image classification. These pre-trained models can be sub-optimal for dense prediction tasks due to the discrepancy between image-level prediction and pixel-level predic
arxiv.org
오랜만에 읽는 SSL 논문이다.
MoCo-v2를 베이스로 하며 MoCo-v2는 image로부터 추출한 하나의 벡터를 기준으로 contrastive learning을 적용한다. 논문에서는 이를 global contrastive learning이라고 언급한다.
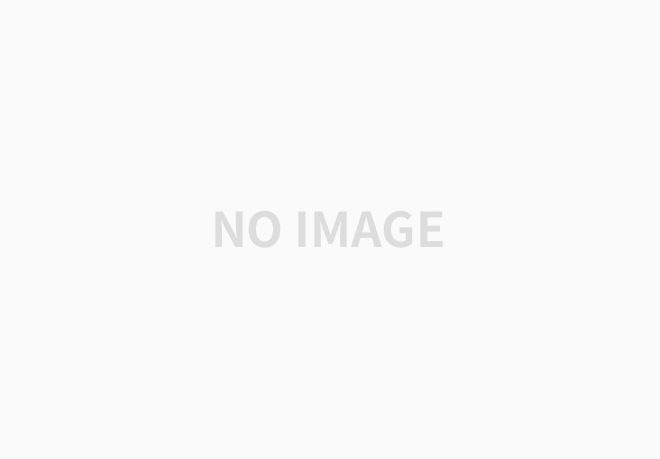
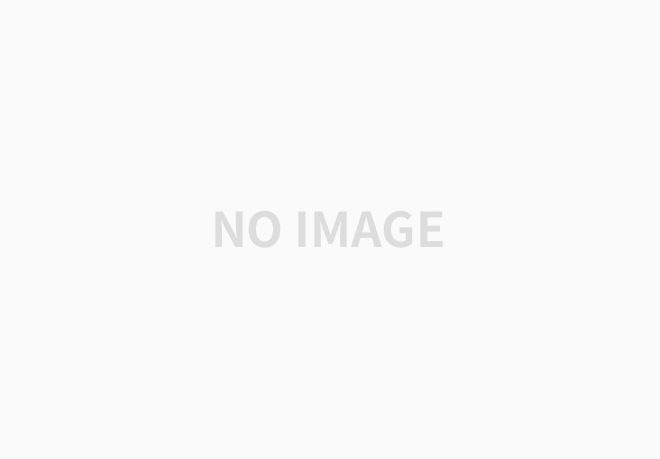
이렇게 학습시킨 모델을 dense prediction이 필요한 task(semantic segmentation, object detection, instance segmentation)에 fine-tuning하면 gap이 존재한다. moco는 image classification을 기준으로 global represenation을 추출해 contrastive learning을 수행했기 때문.
이 gap을 채우기 위하여 dense contrastive learning을 도입한다.
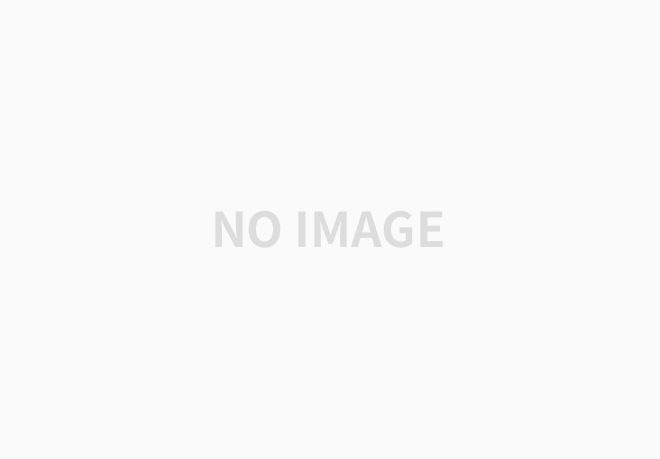
head를 하나 더 추가하여 dense feature map을 추출하고 이 feature map의 vector 사이에 contrastive learning을 수행함.
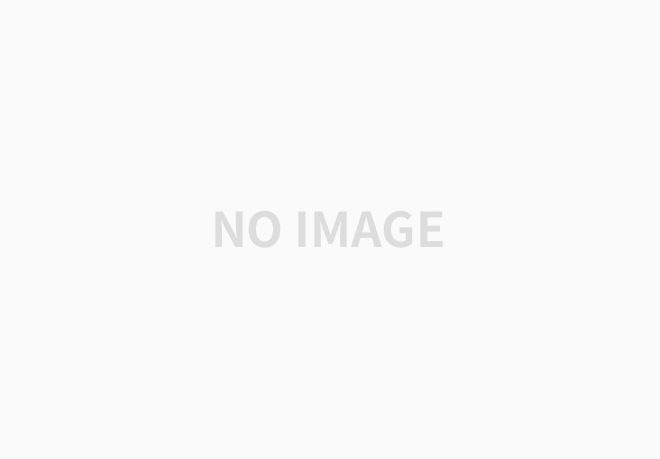
전체 loss는 global contrastive loss와 dense contrastive loss를 함께 사용한다.
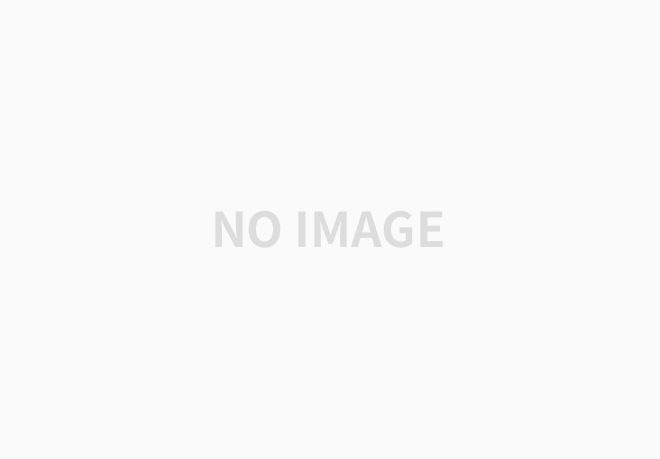
이렇게 학습한 모델은 dense prediction이 필요한 downstream task에 좋은 성능을 보인다. 심지어 supervision을 뛰어넘음.
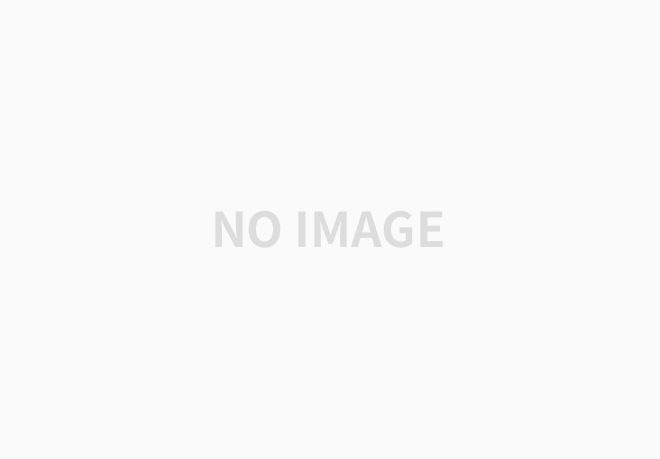
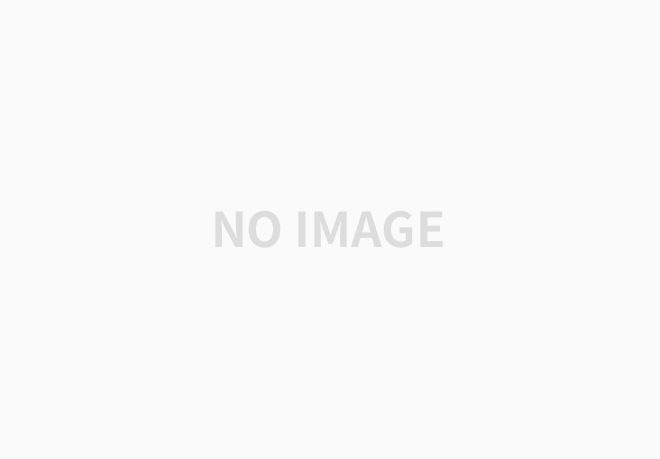
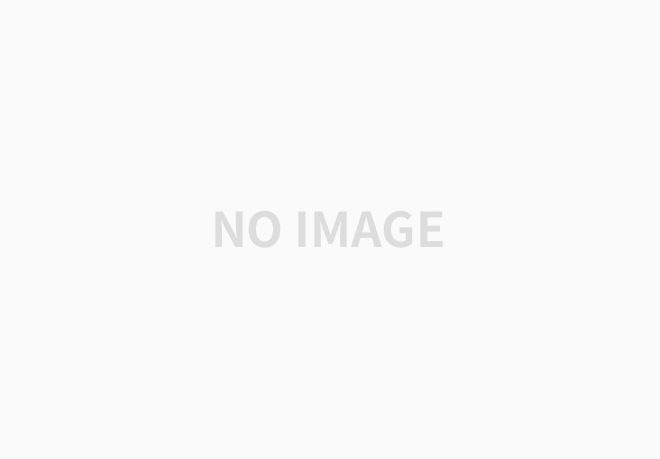
image classification에 대한 성능은 안나와있따. task에 알맞는 방법으로 pre-training을 해야 한다는 건데// 이전 방법들은 ssl 방식으로 image classification 성능을 따라잡는데 집중한 반면에, 이 논문은 task도 고려함
GitHub - Seonghoon-Yu/AI_Paper_Review: 논문을 읽고 블로그에 정리합니다. 정리한 내용을 공유합니다.
논문을 읽고 블로그에 정리합니다. 정리한 내용을 공유합니다. Contribute to Seonghoon-Yu/AI_Paper_Review development by creating an account on GitHub.
github.com